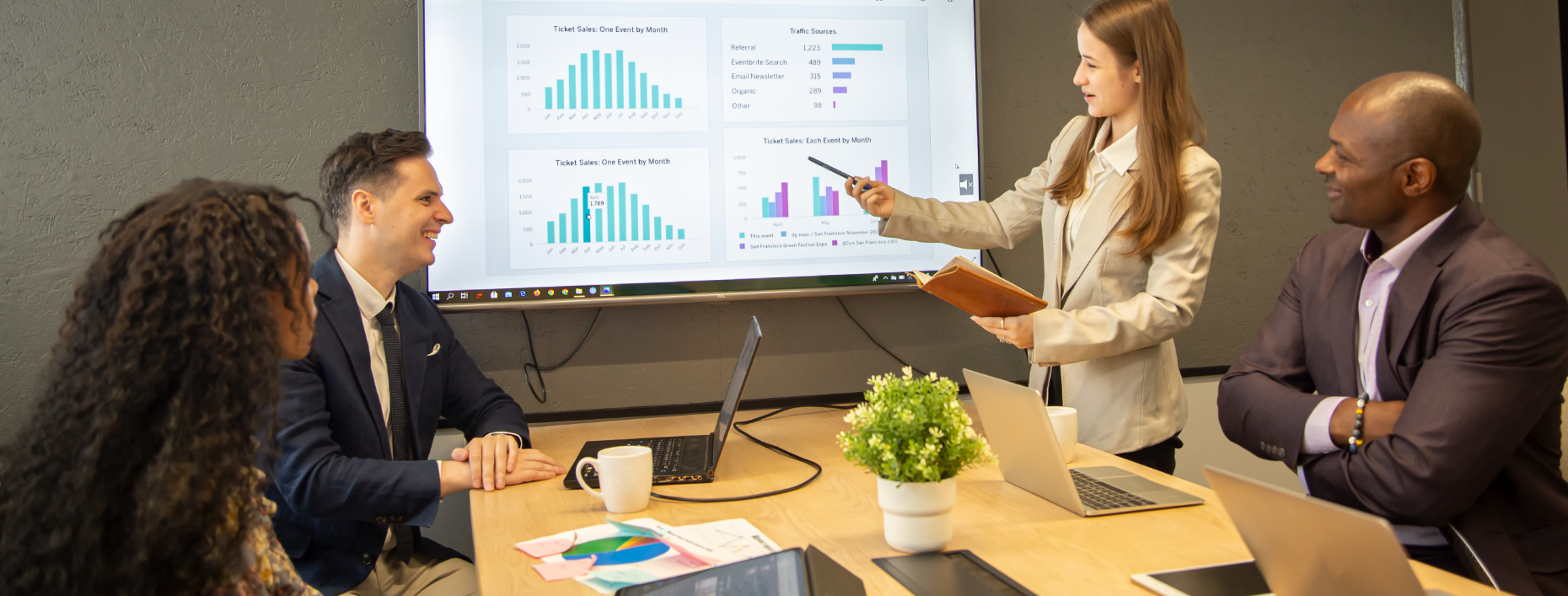
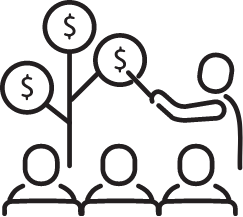
To bring together researchers working on all aspects of risk management to provide innovative solutions to pressing risk management problems. The Risk Intelligence and Resilient Solutions sub-cluster aims to foster rigorous and relevant research with the goal of developing new knowledge and insights into risk management. This would form the basis for the group to make recommendations to relevant parties including regulators, industry organizations and firms in Canada, and government officials.
Navigating Through an Ever-Changing Global Landscape
One of the biggest challenges for businesses in the past decade has been navigating through uncertainty in an unstable and unpredictable economy. While the earlier crises such as the 2008 global financial meltdown had fundamentally changed how many businesses operate, the ongoing COVID-19 pandemic has changed lives, and forced all businesses and individuals to adapt to a new normal. It is in these uncertain times that people realize the fragility of the existing system and the scarcity of resources. A shortage of capital led to the bankruptcy of a bank whereas a shortage of medical equipment led to the loss of lives.
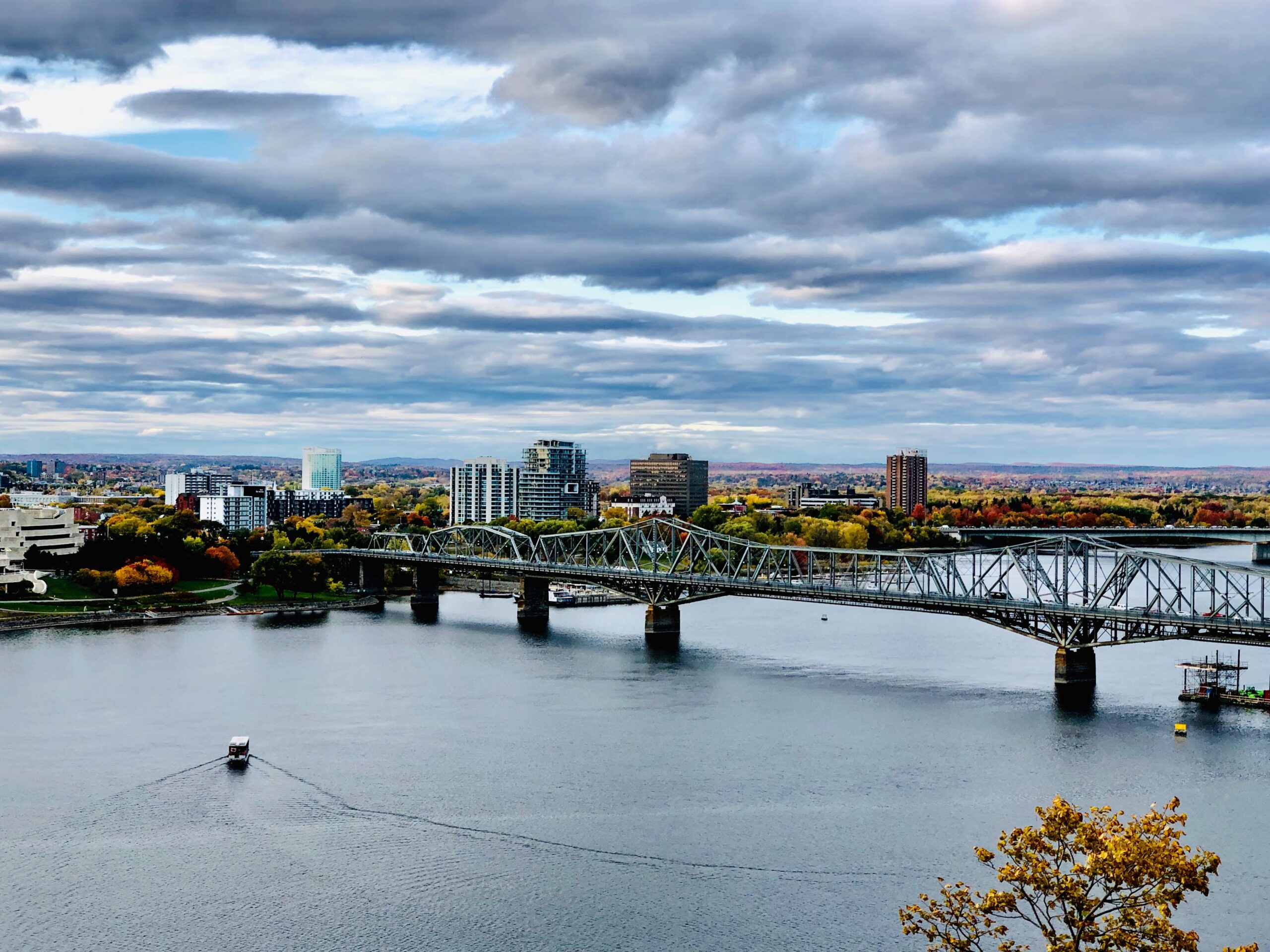
Managing Unknowns
The difficulties of managing uncertainty can be summarized by the term VUCA, an acronym first introduced by United States Army War College and stands for: Volatility – change is rapid and unpredictable in its nature and extent; Uncertainty – the “known unknowns”; Complexity – many interconnected parts and variables; Ambiguity – the “unknown unknowns”. It is now imperative for businesses to sort through these difficulties and learn to manage them.
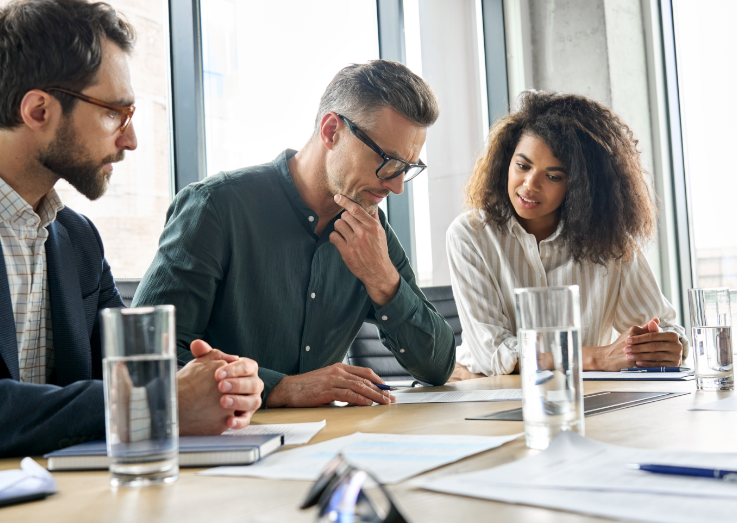
Risk Intelligence
Advance has been made in risk intelligence and tools have been developed for identifying, measuring, and mitigating risk resulting from various sources of uncertainties. Significant progress has been made in improving the reliability of risk measurement using advanced analytics. Indeed, these methodological advancements have made it possible to identify the weakness within Basel 2, a previous prevailing international regulatory framework, and the way larger international banks managed market, credit, and operational risk. They revealed, for instance, that the proposed regulations fail to consider the fact that risk is endogenous and that Value-at-Risk (VaR), the industry-standard measurement of risk, can in fact destabilize an economy and induce crashes.
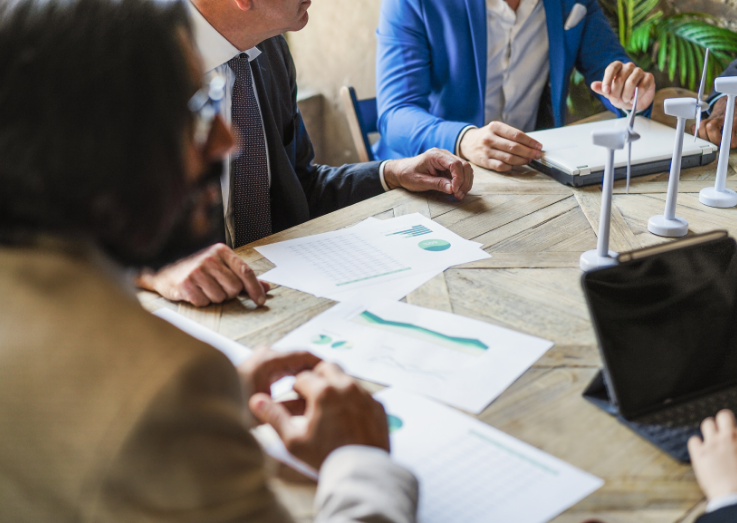
Solutions Powered by Insightful Data and Analytics
Businesses tend to become paralyzed when recognizing the depth and complexity of the uncertainty they are facing. It takes an understanding of the fundamental risk management principles such as diversification and hedging to navigate through the complexity. It also requires the development of a systematic approach that can effectively incorporate the dynamics of uncertain factors into a real-time decision-making process. This has led to the introduction of the framework of enterprise risk management (ERM). One of the key drivers behind the success of this development is today’s ability to model complex decision-making problems. To name a few, models for financial portfolio management have enabled the construction of portfolios that optimize risk-return trade-offs; models for supply chain management have enabled the adjustment of a supply chain that minimizes the disruption risk. Many of these models are made possible by the theoretical and algorithmic advances of optimization, statistics, and financial econometrics in the past decade. Emerging technology such as AI and machine learning also offers opportunities to develop new tools for enhancing risk intelligence.
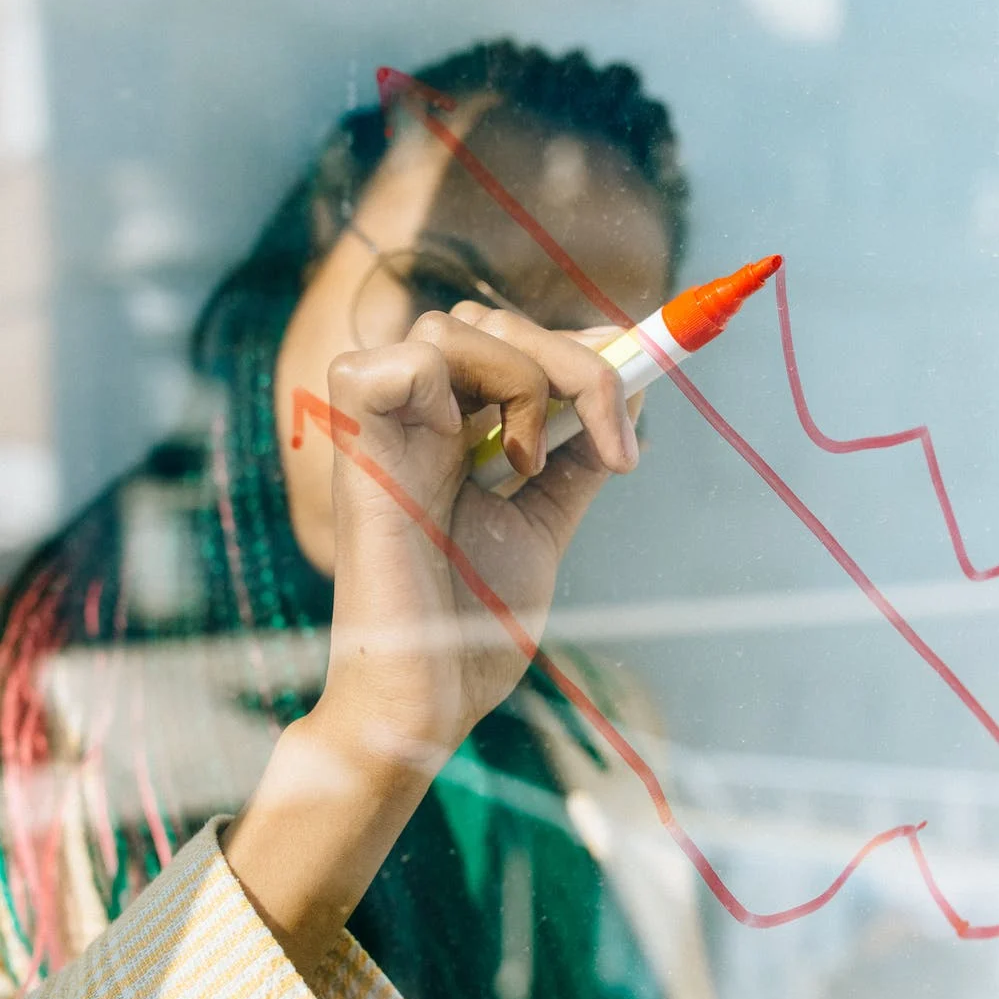
Selection of Relevant Work
Banerjee, P., Dutta, S., 2022
“The Effect of Political Risk on Investment Decisions”, Economic Letters.
Marzban, S., Delage, E., Li, J.Y., 2022
“Equal risk pricing and hedging of financial derivatives with convex risk measures “, Quantitative Finance, 22(1), 47-73.
Racicot, F.E. and Théoret, R., 2022
“Tracking market and non-traditional sources of risks in procyclical and countercyclical hedge fund strategies under extreme scenarios: a nonlinear VAR approach”. Financial Innovation, 8(24): 1-56.
Gregoriou, G.N., Racicot, F.E. and Théoret, R., 2021
“The response of hedge fund tail risk to macroeconomic shocks: A nonlinear VAR approach”, Economic Modelling, 94: 843-872.
Li, J.Y., 2021
“Inverse optimization of convex risk functions “, Management Science, 67 (11): 6629-7289.
Qi, Q(C). and McConnell, J., 2021
“Does CEO Succession Planning Create Shareholder Value?”, Journal of Finance and Quantitative Analysis.
Sun, F., Dutta, S., Zhu, P., Ren, W., 2021
“Female insiders’ ethics and trading profitability”, International Review of Financial Analysis, pp. 1- 18, Volume 74.
Bowen, R., Dutta, S., Tang, S., Zhu, P., 2018
“Inside the ‘Black Box’ of Private In-House Meetings”, Review of Accounting Studies, 23(2):487-527.
Delage, E., Li, J.Y., 2018
“Minimizing risk exposure when the choice of a risk measure is ambiguous”, Management Science, 64(1):327-344, May.
Li, J.Y., 2018
“Closed-form solutions for worst-case law invariant risk measures with application to robust portfolio optimization”, Operations Research, 66(6):1457-1759.
Racicot, F.E., Rentz, W.F. and Théoret, R., 2018
“Testing the new Fama and French factors with illiquidity: A panel data investigation.”, Finance, 39(3): 45-102.
Racicot, F.E., Théoret, R., 2016
“Macroeconomic shocks, forward-looking dynamics, and the behavior of hedge funds.”, Journal of Banking & Finance, 62: 41-61.
Racicot, F.E., 2015
“Engineering robust instruments for GMM estimation of panel data regression models with errors in variables: a note.”, Applied Economics, 47(10): 981-989.
Risk Intelligence and Resilient Solutions Sub-Cluster Coordinator
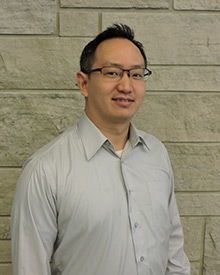
Jonathan Yu-Meng Li, PhD